会议时间:3月21日星期二 8:00-9:30
会议地址:腾讯会议:577-783-671
报告人简介:
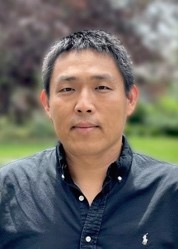
马勤,美国俄亥俄州立大学长聘副教授,生物医学信息学学院生物信息学和计算生物学方向负责人,俄亥俄州立大学综合癌症中心Pelotonia免疫肿瘤学研究所免疫肿瘤学信息学组负责人。在山东大学获得运筹学博士学位,之后在美国佐治亚大学进行博士后研究,专注于高通量测序数据挖掘和建模。之后作为独立PI加入南达科他州立大学,并建立了生物信息学实验室,开始从事单细胞测序数据分析领域的研究。目前,他的实验室专注于开发计算方法,从单细胞多组学数据中发现异质转录调控机制,尤其将相关深度学习方法应用于癌症研究中。在Nature Reviews Molecular Cell Biology、Nature Neuroscience、Nature Microbiology、Nature Communications、Nucleic acids research 等杂志上发表论文100余篇。
实验室网址: https://u.osu.edu/bmbl/.
报告题目: Graph representation learning of single-cell omics data
报告摘要:
Artificial intelligence (AI) and single-cell studies have been making waves in the science and technology communities. AI offers a broad range of methods that can be used to investigate diverse data- and hypothesis-driven questions in single-cell biology (Ma, Q., Xu, D. Deep learning shapes single-cell data analysis. Nat Rev Mol Cell Biol, 2022). The highly heterogeneous nature of single-cell data can be analyzed across a wide range of research topics by generalizing deep-learning model design and optimization in a hypothesis-free manner. This talk will introduce in-house graph representation learning methods for single-cell omics data to discover underlying mechanisms in diverse biological systems.